Reinventing Credit Risk with AI: A Banking Transformation
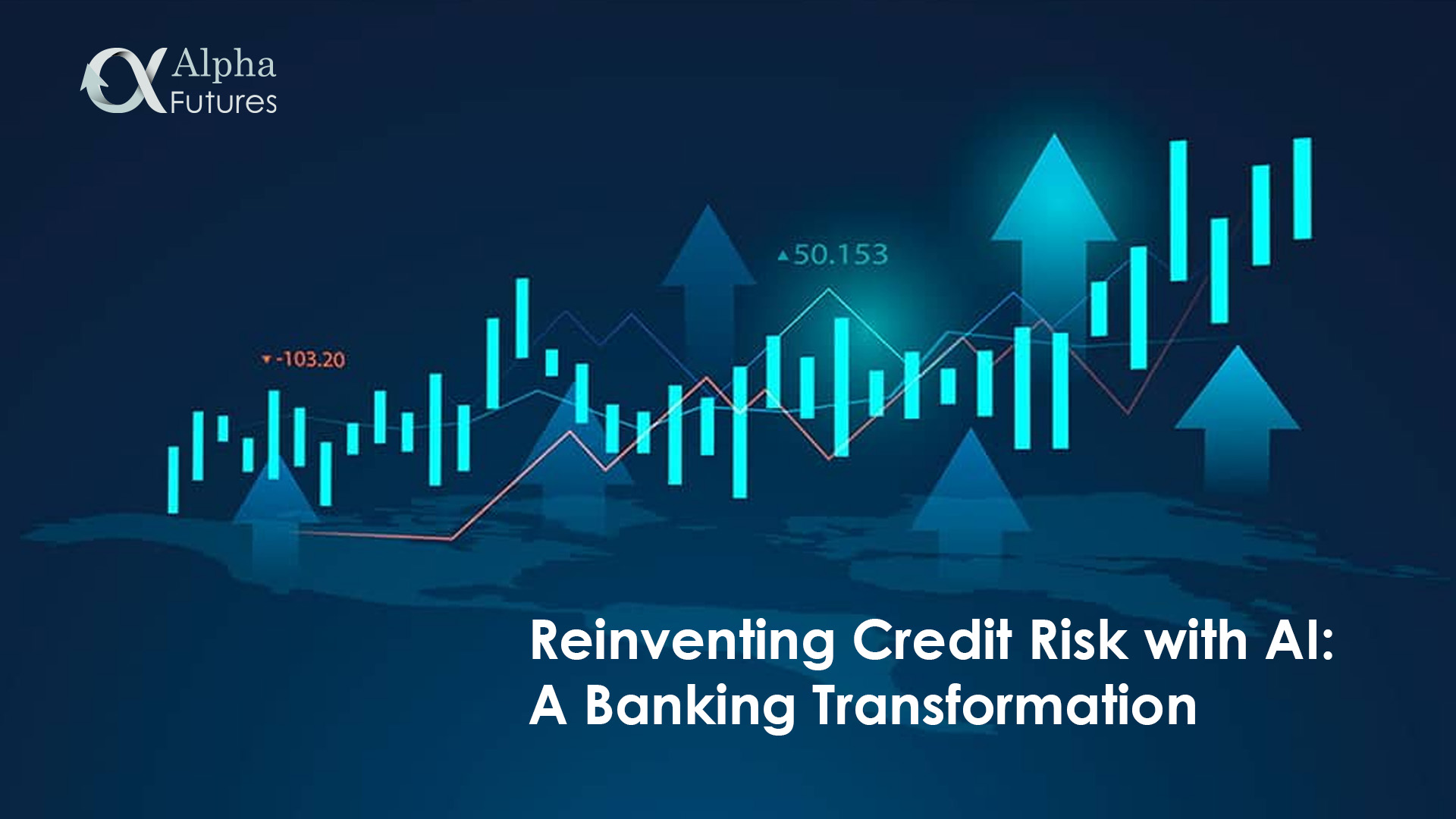
In the dynamic financial landscape, a prominent U.S. bank confronted a critical challenge: its credit risk modelling system was outdated, and inefficient, and struggled to scale with its growing customer base. Manual data analysis procedures proved time-consuming and lacked the precision required in the modern data-driven banking environment. It became evident that a more sophisticated, accurate, and efficient solution was imperative to handle the escalating volume and complexity of Big Data.
Business Objective:
The primary goal was a comprehensive overhaul of the credit risk assessment framework, aiming for higher accuracy and efficiency. Faced with the limitations of the existing semi-manual system, the objective was to implement a state-of-the-art, fully automated, machine learning-based model.
This advanced model would handle vast amounts of data, enabling comprehensive analysis and providing real-time predictive insights to enhance credit decision-making processes and optimize risk management strategies.
How We Achieved It:
Deep Dive and Identification: Commenced with a deep dive into the existing credit risk model to identify areas for AI integration. Sophisticated Machine Learning Tools: Deployed Python, TensorFlow, and Keras to craft a predictive model capable of agile and accurate analysis of vast datasets.
Big Data Handling: Embraced the challenge of Big Data using PySpark and Hadoop for seamless data analysis and processing, with Hive and SQL for effective data warehousing and complex querying tasks.
Robust Technical Architecture: Implemented a robust technical architecture, including data preprocessing, feature engineering, and model training to discern subtle patterns indicative of credit risk.
Meticulous Deployment: Deployed the model into the bank’s operational environment for real-time insights, followed by Tableau dashboards for stakeholder monitoring and strategic adjustments. Identify and implement AI strategies and enterprise-grade solutions with the highest business impact.
The Results:
The transformation was profound, marking a leap forward in the bank’s credit risk management capabilities. Manual processing time saw a stark reduction, freeing up resources and lowering operational costs. Predictive accuracy soared, resulting in a remarkable 20% reduction in losses and default rates.
Credit decisions became dynamic and responsive, driven by real-time insights for proactive risk management. Tangible outcomes included improved loan quality, optimized interest margins, and an overall healthier portfolio. These improvements led to greater investor confidence and a strengthened market position.
As the bank leveraged AI-driven insights, it refined credit policies, tailored product offerings, and enhanced customer satisfaction through personalised and efficient service. Beyond savings, these improvements represented revenue growth opportunities, allowing the bank to confidently expand its credit offerings with risk managed by sophisticated AI tools.